Imagine a canvas that breathes life into its creations, a melody that resonates with the very essence of your soul, or a story that unfolds in ways you never anticipated. This is the captivating world of Generative Adversarial Networks (GANs), where the fusion of human imagination and machine intelligence pushes the boundaries of artistic expression.
From the mesmerizing video installations of Refik Anadol that blur the lines between light and shadow to the song “Daddy’s Car” featuring AI-generated soundscapes, GANs are empowering both visual artists and musicians to explore new creative possibilities and collaborate in innovative ways.
Conceived by Ian Goodfellow and his colleagues in 2014, GANs have since become the cornerstone of modern artificial intelligence. The concept behind GANs is elegant in its simplicity: two neural networks, known as the generator and the discriminator, engage in a perpetual game of cat and mouse.
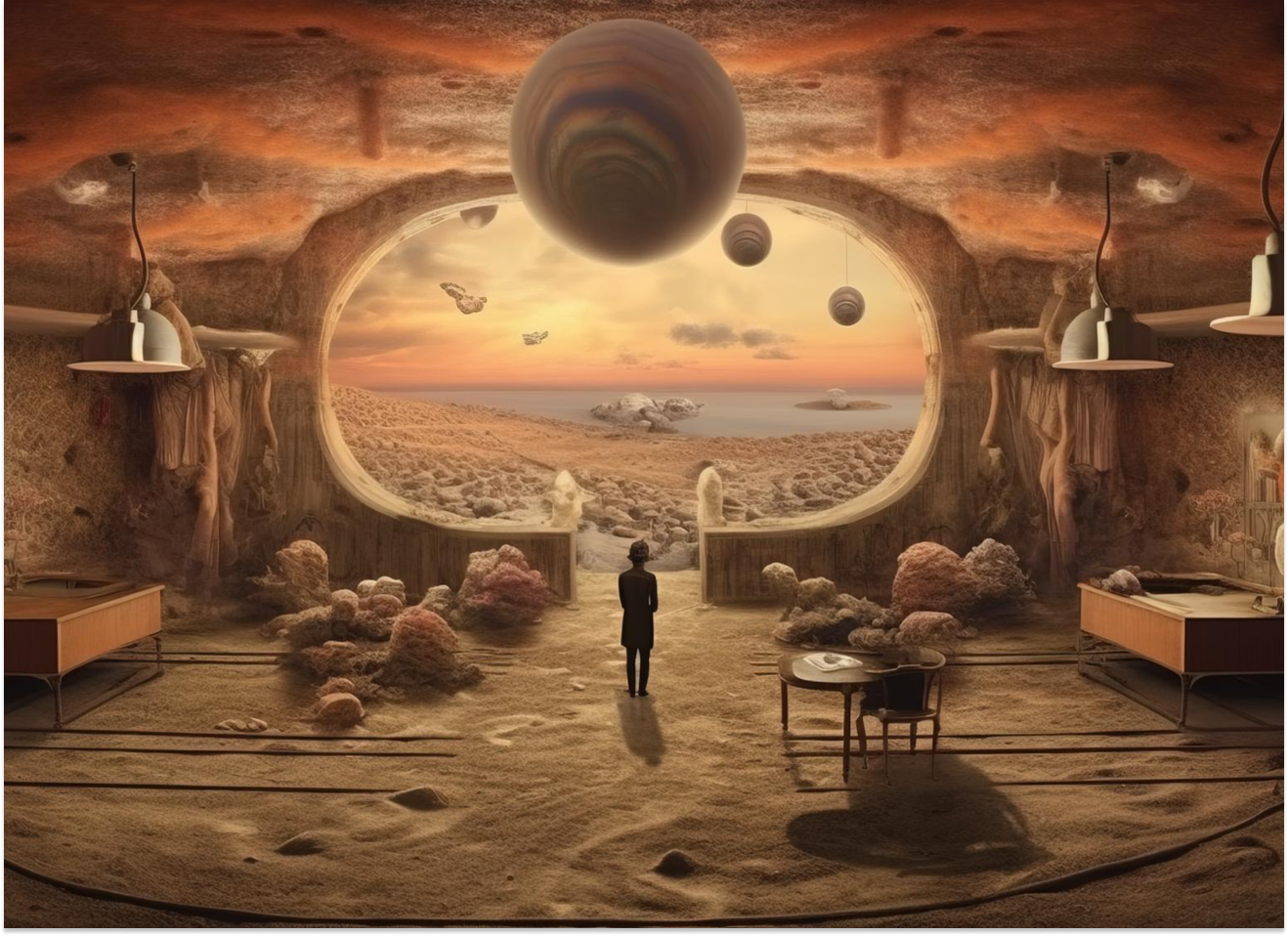
"Inside the Creative Clash of Generators and Discriminators"
In this captivating interplay, the two neural networks, the generator and the discriminator, engage in a perpetual dialogue, each striving to outwit and inspire the other.
The generator’s mission is to produce images, sounds, or text that are indistinguishable from real data in a given domain. It starts with random noise as its raw material and gradually refines it through a series of transformations, learning to produce output that increasingly resembles genuine artwork.
Meanwhile, the discriminator acts as the discerning eye, tasked with differentiating between real and generated samples. It scrutinizes each creation from the generator, providing feedback on its authenticity. Through this process, the discriminator hones its ability to distinguish between genuine and synthetic content.
As the training progresses, the generator and discriminator engage in a delicate dance of one-upmanship. With each iteration, the generator strives to produce a more convincing output to fool the discriminator, while the discriminator becomes increasingly adept at spotting subtle flaws in the generated samples.
The true magic of GANs lies in their adversarial nature – the competition between the generator and discriminator drives them to continuously improve, resulting in outputs that are remarkably close to reality. This adversarial training process allows GANs to capture the intricate details, textures, and nuances that make art so captivating. But GANs are not limited to replicating existing art – they can also push the boundaries of creativity by exploring uncharted territories.
By manipulating the input noise or adjusting the network architecture, artists and researchers can steer the GAN towards producing entirely new and unexpected forms of expression.
Amidst the awe-inspiring potential of GAN-generated art lies a profound question: where does the line between human and machine creativity truly lie? As we marvel at the masterpieces created by GANs, we are forced to confront the limits of our imagination and the implications of a world where machines are capable of producing art that rivals our own. In this ever-evolving landscape of art and technology, one thing is certain: the magic of GAN-generated art is only just beginning.
“Even if we do not talk about 5G (specifically), the security talent in general in the country is very sparse at the moment. We need to get more (security) professionals in the system”